Unleashing the Power of Machine Learning for Control Systems
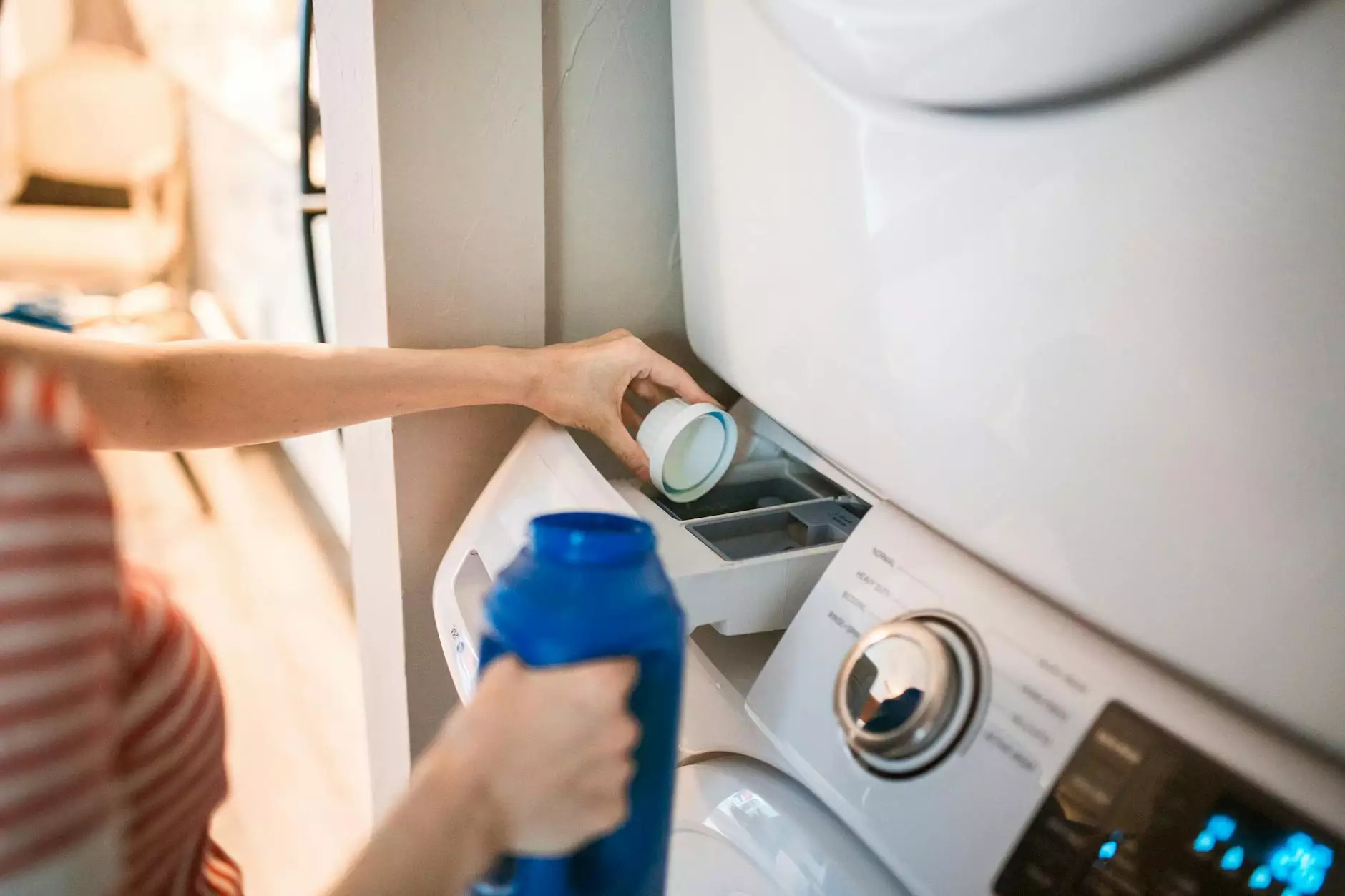
Machine learning has emerged as a transformative force in various sectors, and its integration into control systems is a prime example of how businesses can leverage advanced technology to streamline operations and enhance decision-making. In this article, we will explore the significance of machine learning for control systems, examining its applications within the realms of content management services, business process automation, and data governance systems.
The Intersection of Machine Learning and Control Systems
At its core, the concept of machine learning involves the use of algorithms and statistical models by computer systems to perform specific tasks without explicit instructions. This ability to learn from data presents an unprecedented opportunity to optimize control systems, which are critical for many business processes ranging from supply chain management to customer relationship management.
What are Control Systems?
Control systems are essential frameworks designed to manage, command, direct, or regulate the behavior of other devices or systems. In the context of business, these can include processes, machinery, and entire business operations. By integrating machine learning for control systems, organizations can achieve higher efficiency, accuracy, and adaptability to changing environments.
Why Implement Machine Learning in Control Systems?
- Enhanced Predictive Capabilities: Machine learning algorithms can analyze historical data to predict future outcomes, improving decision-making processes.
- Real-Time Adjustments: With continuous learning capabilities, control systems can adjust parameters in real-time, mitigating disruptions and optimizing performance.
- Data-Driven Insights: Machine learning provides valuable insights that help organizations refine their strategies and operations.
- Cost Reduction: Automated processes reduce manual intervention, lower operational costs, and enhance overall efficiency.
Exploring Applications in Business
The implications of machine learning for control systems stretch across various business categories, notably in content management services, business process automation, and data governance systems.
1. Content Management Services: Transforming Information Flow
In the digital age, effective content management is paramount for any organization. Businesses need robust systems that not only store but also intelligently manage content. Machine learning enhances content management by enabling systems to:
- Automate Categorization: Automatically tagging and organizing content based on context and relevance.
- Improve Searchability: Utilizing natural language processing (NLP) to provide better search results based on user queries.
- Personalize User Experience: Analyzing user behavior to customize content delivery and recommendations.
- Streamline Workflows: Identifying bottlenecks in content workflows and suggesting optimization strategies.
2. Business Process Automation: Maximizing Efficiency
Business process automation (BPA) has become essential for organizations looking to enhance productivity and reduce costs. Incorporating machine learning for control systems offers various advantages:
- Enhanced Decision-Making: Automation systems backed by machine learning can analyze vast datasets to inform better business decisions.
- Predictive Maintenance: Anticipating equipment failures through predictive analytics, leading to timely maintenance and reduced downtime.
- Process Optimization: Continuously learning from process data to identify inefficiencies and recommend improvements.
- Scalability: Automated processes can be easily scaled up or down based on business demands, ensuring flexibility.
3. Data Governance Systems: Ensuring Compliance and Integrity
In today's data-driven environment, data governance is critical for maintaining data quality, security, and compliance with regulations. Machine learning enhances data governance systems by:
- Real-Time Monitoring: Continuously checking data entries and usage for compliance and quality assurance.
- Automated Reporting: Generating compliance reports without manual effort, saving time and reducing errors.
- Risk Assessment: Identifying potential data governance risks through historical analysis and predictive capabilities.
- Data Lineage Tracking: Monitoring the origin of data, its movement, and transformations across systems for transparency.
Challenges and Considerations
While the advantages of machine learning for control systems are substantial, organizations must also consider several challenges when implementing these technologies:
- Data Quality: Machine learning algorithms require high-quality data for effective performance. Poor data quality can lead to erroneous outputs.
- Complex Integration: Integrating new machine learning systems with existing technologies can pose significant challenges.
- Change Management: Employees may need training to adapt to new technologies, which can be a time-consuming process.
- Security Concerns: As with any technology, machine learning systems can be vulnerable to security breaches, requiring robust safeguards.
The Future of Machine Learning for Control Systems
The future of business heavily relies on the integration of technologies like machine learning for control systems. As businesses continue to adapt to technological advancements, those that embrace machine learning will likely lead their industries. Future trends might include:
- Increased Automation: A gradual shift towards more sophisticated automation tools that require minimal human intervention.
- Edge Computing: Moving machine learning processes closer to where data is generated, allowing for faster decisions and reduced latency.
- Enhanced AI Collaboration: As AI technologies improve, their ability to collaborate with human intelligence will create more dynamic and efficient business environments.
- Focus on Ethics: As organizations navigate the complexities of AI, there will be an increased emphasis on ethical considerations in machine learning applications.
Conclusion
In conclusion, the application of machine learning for control systems represents a monumental shift in the way businesses operate. By harnessing this technology, organizations can improve efficiency, enhance decision-making, and maintain competitive advantages across industries. As we continue to embrace advancements in machine learning, the possibilities for innovation and growth will only expand, making it an essential element in the future of business strategy.