The Power of a Medical Dataset for Machine Learning in Healthcare
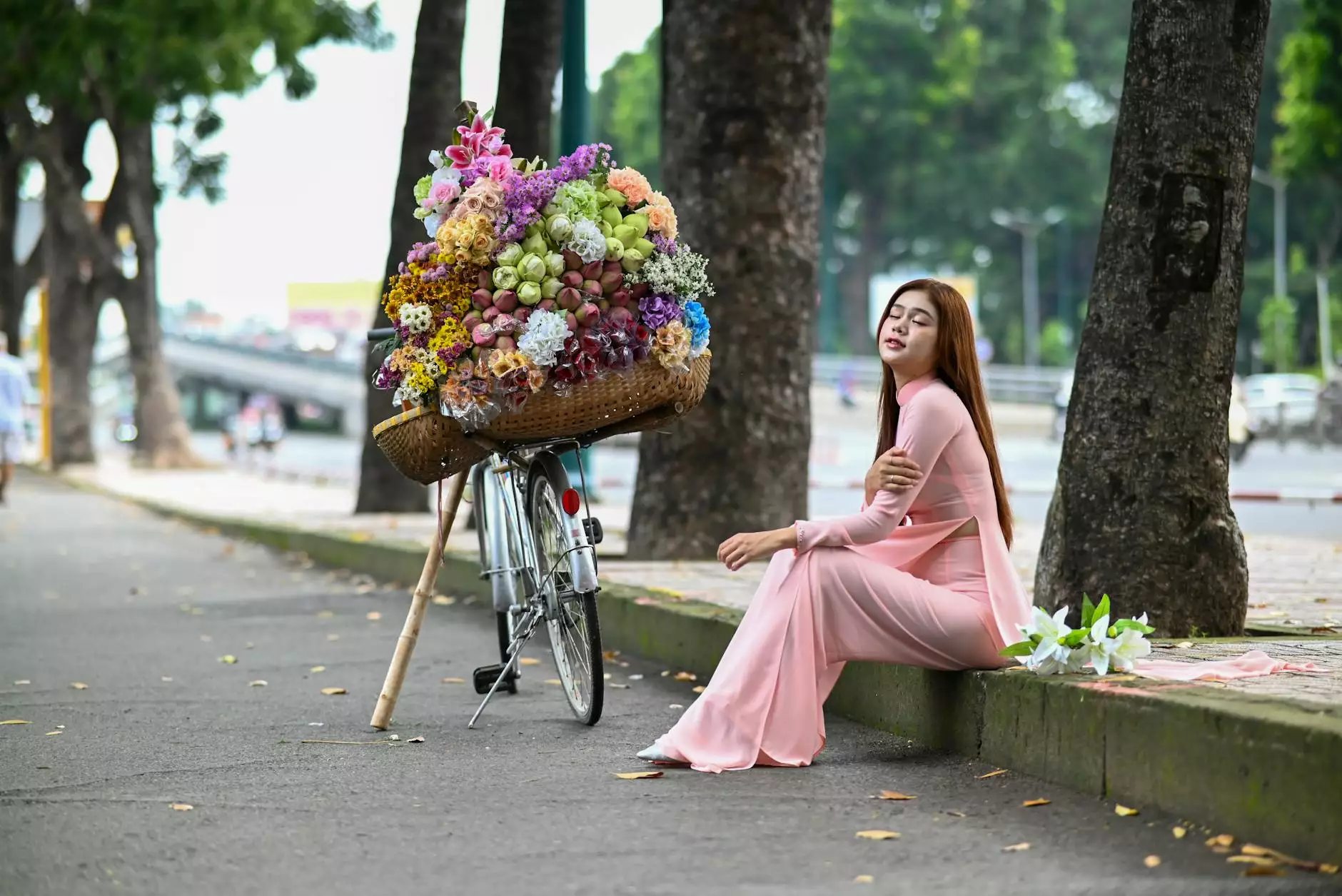
In today's rapidly evolving healthcare landscape, the integration of technology and artificial intelligence is playing a pivotal role in enhancing patient care. A medical dataset for machine learning has emerged as one of the most critical tools that healthcare professionals and researchers utilize to unlock insights that were previously unimaginable. This article will delve deep into the significance of medical datasets, their applications in machine learning, and the transformative effects they could have on modern healthcare.
Understanding Medical Datasets
Medical datasets are collections of data that often encompass various aspects of patient health, diagnosis, treatment history, and outcomes. These datasets can vary widely based on their source, type of data, and the specific healthcare domain they address. Common types of data found in medical datasets include:
- Electronic Health Records (EHRs): Comprehensive patient records that contain demographics, medical history, medications, allergies, lab results, and more.
- Clinical Trials Data: Information from clinical studies that detail participants' treatments, responses, and outcomes.
- Medical Imaging Data: Images derived from MRI, CT scans, X-rays, and ultrasound scans that provide visual insights into patient health.
- Genomic Data: Information that relates to the genetic makeup of patients, which can provide insights into disease susceptibility and treatment responses.
- Wearable Device Data: Data generated from fitness trackers and medical monitoring devices that continuously monitor patient health metrics.
Why is a Medical Dataset Essential for Machine Learning?
The incorporation of machine learning in healthcare offers vast potential to improve efficiency, accuracy, and patient outcomes. However, for machine learning algorithms to be effective, they require vast amounts of quality data. A medical dataset for machine learning serves several crucial functions:
1. Enhancing Diagnostic Accuracy
Machine learning models trained on comprehensive medical datasets can assist healthcare providers in diagnosing diseases with higher accuracy. These algorithms can analyze patterns within vast amounts of data, identifying correlations and risk factors that human practitioners might overlook. For instance, a model could automatically analyze imaging data to detect early signs of cancer, significantly increasing the chances of successful treatment.
2. Personalizing Treatment
By leveraging a medical dataset for machine learning, healthcare professionals can tailor treatments to individual patient needs. Machine learning helps in predicting how different patients will respond to various treatments based on their unique health profiles. Personalized medicine not only enhances treatment efficacy but also minimizes the risk of adverse effects, leading to better patient outcomes.
3. Predictive Analytics for Patient Outcomes
Predictive analytics uses historical data to forecast future events. In the healthcare context, machine learning models can predict potential complications or readmission risks. By analyzing patient records, these models can flag patients who might benefit from additional monitoring or intervention, thereby improving overall healthcare efficiency.
4. Operational Efficiency
Medical datasets enable healthcare facilities to optimize their operations. By analyzing patient flow and resource utilization, machine learning algorithms can provide insights that lead to better staffing decisions, reduced wait times, and enhanced patient satisfaction. Streamlining operations directly correlates to better care and improved financial performance for healthcare providers.
Challenges in Leveraging Medical Datasets
Despite the undeniable benefits, there are significant challenges associated with utilizing medical datasets for machine learning, including:
- Data Privacy and Security: Protecting patient data is paramount, and healthcare providers must navigate complex regulations such as HIPAA to ensure compliance.
- Data Quality and Integrity: The effectiveness of machine learning models is heavily dependent on the quality of the input data. Inaccuracies or inconsistencies in datasets can lead to misleading conclusions.
- Interoperability: Different healthcare systems often use varying formats and standards for data. Ensuring that datasets can work together seamlessly is a significant hurdle.
- Bias in Data: If a dataset is not representative of the entire population, it can lead to biased outcomes. Ensuring diversity in datasets is essential for fairness and accuracy.
How to Acquire and Utilize Medical Datasets for Machine Learning
Acquiring a quality medical dataset for machine learning involves several steps:
1. Identifying the Right Dataset
Depending on the research or application focus, identifying the right dataset is crucial. Researchers can seek publicly available datasets, collaborate with healthcare institutions, or generate proprietary datasets through studies.
2. Ensuring Compliance and Ethical Use
It is vital to ensure that all datasets are acquired and used in compliance with legal standards and ethical guidelines, particularly regarding informed consent from patients whose data is included.
3. Data Preprocessing
Once a dataset is acquired, it often requires cleansing and preprocessing. This step involves removing duplicates, correcting errors, filling in missing values, and transforming data into a suitable format for training machine learning models.
4. Training Machine Learning Models
With a quality dataset at hand, machine learning models can be trained to recognize patterns and make predictions. Various algorithms can be employed, such as decision trees, neural networks, or support vector machines, depending on the specific application.
5. Evaluating and Refining Models
After training, it's essential to evaluate the performance of machine learning algorithms using test datasets. Metrics such as accuracy, precision, recall, and F1 scores should be analyzed to gauge effectiveness. Continuous refinement is key to improving model performance over time.
The Future of Machine Learning in Healthcare
As technology continues to advance, the potential impact of medical datasets for machine learning in healthcare will only grow. Emerging trends and innovations that point to this potential include:
- Increased Use of Predictive Algorithms: More healthcare providers will adopt predictive algorithms to anticipate healthcare needs, improving resource allocation.
- Integration of AI with Telemedicine: The combination of AI and telemedicine will enhance remote patient monitoring and virtual consultations, making healthcare accessible to more people.
- Advances in Genomics: As genomic data becomes more accessible, machine learning algorithms will be increasingly used to tailor treatments based on genetic profiles.
- Emergence of Federated Learning: This new approach allows models to learn on decentralized data without the need for data sharing, addressing privacy concerns while improving AI capabilities.
Conclusion
The integration of a medical dataset for machine learning in healthcare is transforming the industry, paving the way for enhanced diagnostic accuracy, personalized treatment, predictive analytics, and operational efficiency. While challenges exist in leveraging these datasets, the potential benefits far outweigh the obstacles. The future of healthcare will heavily rely on data-driven insights, and as technology progresses, we will continue to uncover new ways to improve patient care through the power of machine learning.
As we stand at the cusp of this data revolution, the importance of high-quality medical datasets cannot be overstated. Should you wish to remain at the forefront of this healthcare evolution, it is imperative to understand, acquire, and utilize medical datasets effectively and ethically.